In recent years, the UK social housing sector has faced increasing pressure to optimise its allocation processes. With long waiting lists and limited housing stock, housing associations and local authorities are turning to innovative solutions to address these challenges. Enter big data and predictive analytics – powerful tools that are transforming how social housing is allocated, making the process more efficient, fair, and responsive to tenant needs, as Nick Francis explores in this article.
The Power of Data in Social Housing
Social housing providers collect vast amounts of data on their properties, tenants, and applicants. This information includes:
Demographic details of applicants
Property characteristics and conditions
Tenant histories and preferences
Neighbourhood data and local amenities
Historical allocation patterns and outcomes
Until recently, much of this data remained siloed and underutilised. However, with advancements in data analytics and machine learning, housing providers can now harness this wealth of information to make more informed decisions.
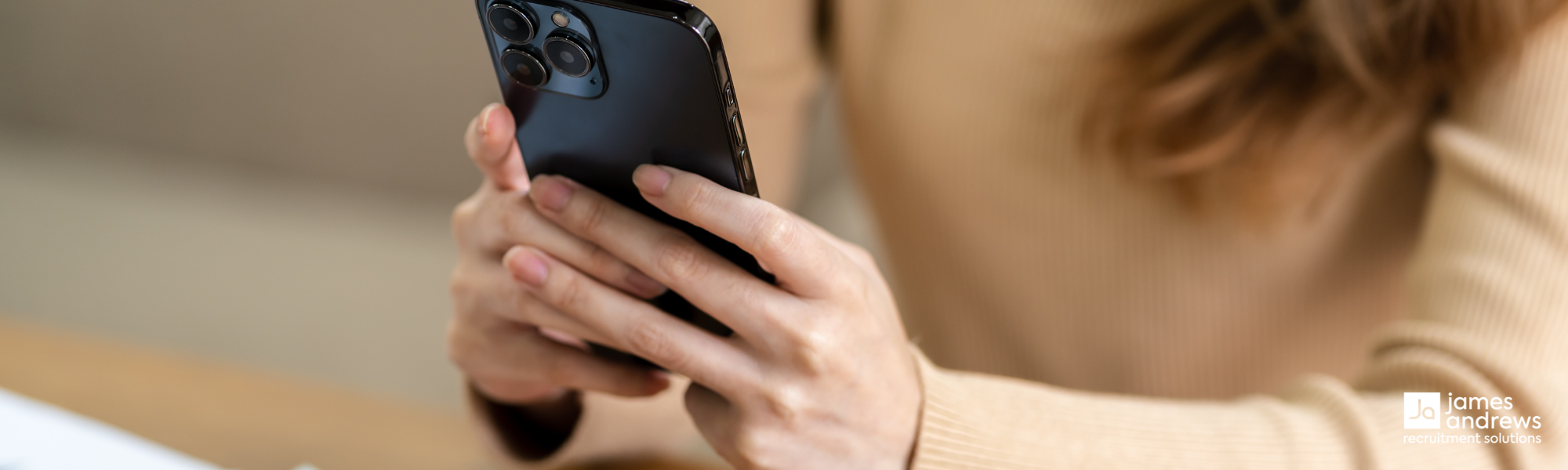.png)
How Predictive Analytics Improves Allocation
Predictive analytics uses historical data to forecast future trends and outcomes. In social housing allocation, this technology is being applied in several ways:
Optimising Tenant-Property Matching: By analysing past successful tenancies, predictive models can suggest matches between available properties and applicants that are likely to result in long-term, stable tenancies.
Anticipating Housing Needs: Data models can predict future housing demands based on demographic trends, allowing providers to plan ahead and adjust their housing stock accordingly.
Reducing Void Periods: Analytics can identify properties at risk of becoming vacant and suggest preemptive actions to minimise void periods.
Prioritising Vulnerable Applicants: Machine learning algorithms can help identify applicants with the most urgent housing needs, ensuring they receive priority in a fair and transparent manner.
Improving Tenant Satisfaction: By analysing factors that contribute to tenant satisfaction, providers can make allocation decisions that are more likely to result in happy, long-term tenancies.
Real-World Impact
While specific, publicly available data on the impact of big data solutions in UK social housing can be limited, there is growing evidence of its potential benefits. Here are some general trends and examples:
Many housing associations report reduced void periods after implementing data-driven allocation systems, though the exact percentages can vary widely.
Improvements in tenancy matching success rates are commonly observed, with some providers noting significant increases in tenant satisfaction and retention.
Several housing authorities have reported reductions in their waiting lists through optimised allocation processes, though the extent of improvement depends on various factors including local housing demand and available stock.
It's important to note that the specific impacts can vary greatly depending on the size of the housing provider, the quality of their data, and the specific solutions implemented. Housing associations interested in adopting these technologies should conduct thorough research and potentially pilot programs to assess the impact in their specific context.
The Role of Data Analysts in Social Housing
As the sector increasingly embraces these technologies, the demand for skilled data professionals is soaring. Key roles include:
Data Scientists: Develop and implement predictive models for housing allocation.
Data Analysts: Interpret complex datasets to provide actionable insights for decision-makers.
Business Intelligence Specialists: Create data visualisation tools and dashboards for easy interpretation of allocation trends.
Database Administrators: Ensure the integrity and security of the vast amounts of sensitive data collected.
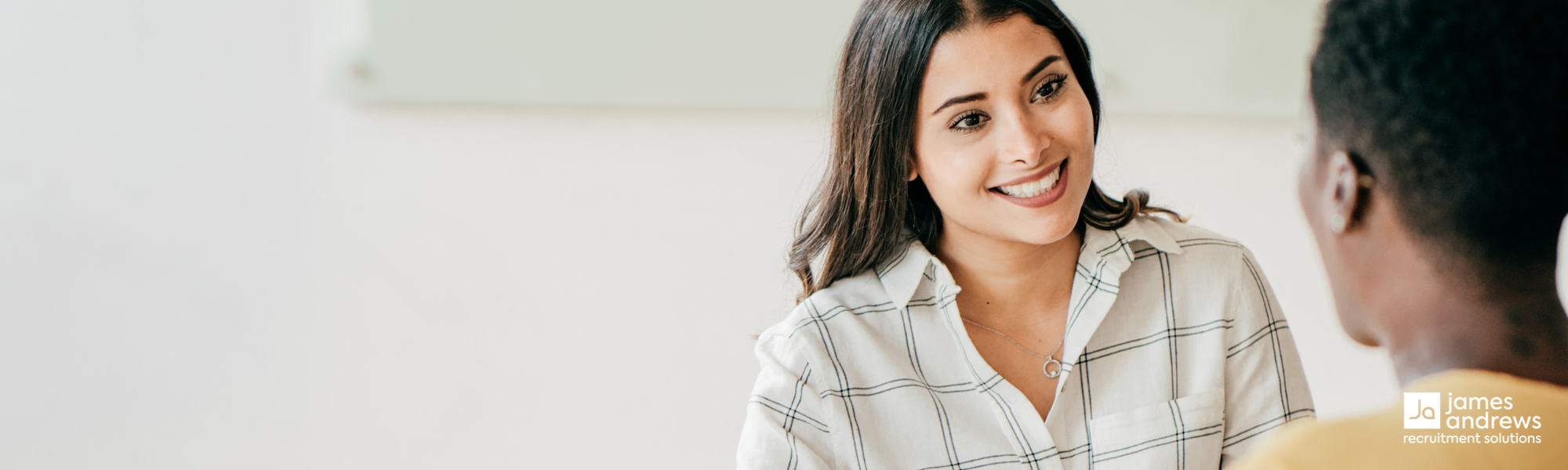.png)
Challenges and Considerations
While the potential of big data in social housing allocation is immense, it's not without challenges:
Data Privacy: Handling sensitive personal information requires robust data protection measures.
Ethical Considerations: There's a need to ensure that algorithms don't inadvertently discriminate against certain groups.
Data Quality: The effectiveness of predictive models relies heavily on the quality and completeness of input data.
Staff Training: Housing professionals need training to effectively use and interpret data-driven insights.
The Future of Data-Driven Allocation
As technology continues to evolve, we can expect even more sophisticated applications of big data in social housing allocation. Machine learning models will become more accurate, potentially incorporating real-time data to make dynamic allocation decisions.
The integration of Internet of Things (IoT) devices in social housing properties could provide a wealth of new data points, further refining the allocation process. For instance, sensors could provide real-time information on property conditions, allowing for proactive maintenance and more accurate matching of tenants to suitable homes.
Conclusion
Big data and predictive analytics are not just buzzwords in the social housing sector – they represent a fundamental shift in how housing is allocated. By leveraging these technologies, housing providers can make more informed decisions, reduce inefficiencies, and ultimately provide better outcomes for tenants.
For professionals in the field, this shift presents exciting opportunities. Data analysts, scientists, and other tech specialists have the chance to make a real difference in addressing one of society's most pressing issues – the provision of quality, affordable housing to those who need it most.
As we move forward, the social housing sector will increasingly rely on data-savvy professionals to drive innovation and efficiency. For those with the right skills, the future is bright in this rapidly evolving field.
With over eight years in technology recruitment, Nick Francis excels in building teams and nurturing client relations across the UK in IT, fintech, and engineering, and is known for strategic improvements and robust client engagement. Nick maintains strong networks in the UK and offers a consultative approach to clients and candidates, with his initiative in implementing process automation, showcasing his ability to blend technical innovation with business strategies. To discuss working with Nick to grow your team, or to find a new role, email nfrancis@jarsolutions.co.uk or schedule a confidential consultation here.